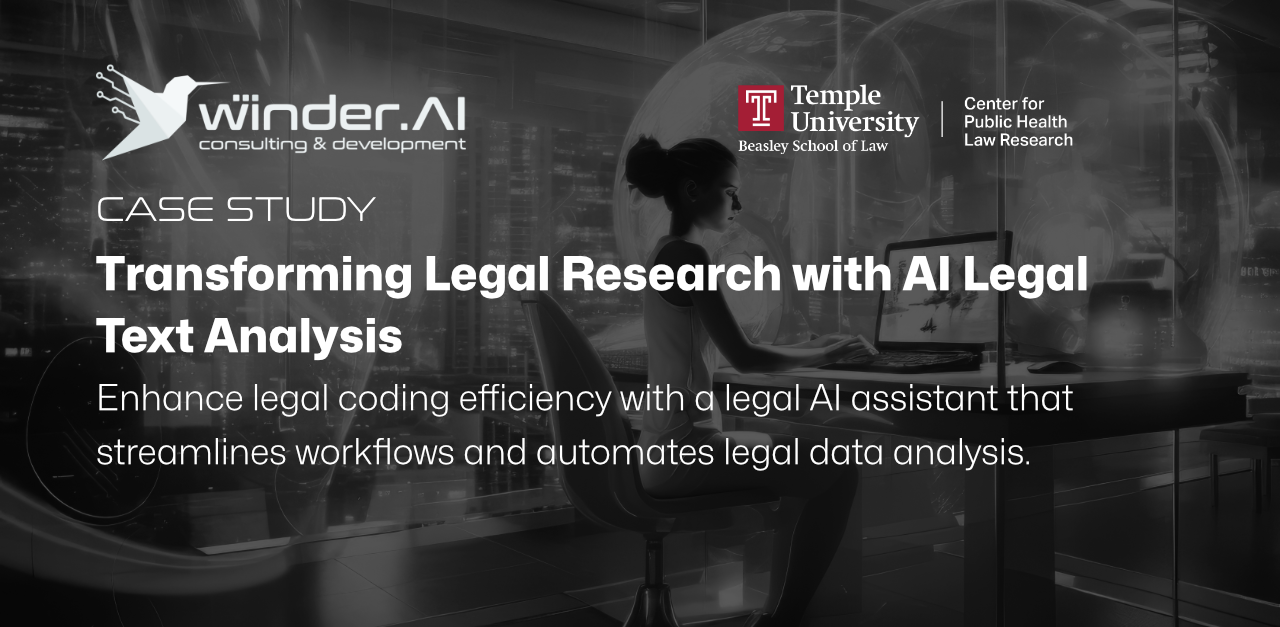
Case study
Transforming Legal Research with AI Legal Text Analysis
Legal AI assistant improves legal coding efficiency by automating data analysis, reducing workload, and accelerating research.
Tired of fragile ML processes? Our MLOps services deliver resilience, automation and unification across your organization.
I would recommend Winder.AI because they are experts with real-world experience, led by Phil Winder, who is well-respected in the industry. They are quick to respond, quick to scale up and they deliver when you need them to.
Our MLOps services bring governance to your AI operations. Scale out, unify, control and fulfil regulatory requirements.
Enterprise MLOps Development Our enterprise MLOps engineers have helped teams scale their machine learning operations. From point improvements to staff augmentation, we’ve got you covered, e.g. Interos.
MLOps Consulting Improving your MLOps maturity helps unify your stack, improve data science efficiency and meet governance requirements, e.g.Tractable.
MLOps Audit Effective change starts with a proactive maturity assessment. By isolating pain points and prioritizing opportunities, an MLOps audit can change your business, e.g. a finance company.
We've worked with hundreds of amazing people, all over the world.
Enterprise-ready Machine Learning Operations solutions.
Unlike larger, more general purpose IT agencies, we specialize in developing AI solutions. We’re more flexible, more pragmatic and better suited to integrate AI into your product.
We combine our bespoke AI solutions with cloud-agnostic or on-premise software engineering to produce production-ready large language models.
We exist to help companies like yours build better products. We collaborate closely with your internal engineering teams to both upskill and deliver pragmatic results.
Winder.AI is a flexible, decentralized, independent AI company that can deliver full-stack solutions. MLOps enables efficient, scalable, predictable AI.
MLOps excels at delivering AI at scale, from massive experimentation to huge deployments.
One major growing pain is the lack of unification between disparate AI teams. Providing a consistent landscape can help improve governance posture and efficiency.
Current and future legislation places requirements on AI systems. MLOps provides the backbone solution with auditing, logging, monitoring and governance.
Make it easier to govern your AI inventory. Catalog, track and promote reproducibility.
Help data scientists be more productive. Parallelize experimentation, compare, time travel and unify.
Scale your data ingestion or training pipelines to meet your demands. Train massive models, make it more repeatable, take advantage of hybrid cloud and modern hardware.
Package models and make deployment a breeze. Auto-deploy to production, serve in a variety of protocols, automatically scale to meet demand, or scale down to save costs.
Maintain your integrity with model monitoring and alerting. Watch for drift, provide analytics, implement continuous learning, alert before catastrophe.
Case study
Legal AI assistant improves legal coding efficiency by automating data analysis, reducing workload, and accelerating research.
Case study
Interos, a leading supply chain management company, partnered with Winder.AI to enhance their machine learning operations (MLOps). Together, we developed advanced MLOps technologies, including a scalable annotation system, a model deployment suite, AI templates, and a monitoring suite. This collaboration, facilitated by open-source software and Kubernetes deployments, significantly improved Interos’ AI maturity and operational efficiency.
Case study
We’re pleased to announce the release of Stable Audio, a new generative AI music service. Stable Audio is a collaboration between Stability AI and Winder.AI that leverages state-of-the-art audio diffusion models to generate high-quality music from a text prompt.
MLOps
Machine learning (ML) model monitoring is a crucial part of the MLOps lifecycle. It ensures that your models are performing as expected and that they are not degrading over time. There are many tools available to help you monitor your models, from open-source frameworks to proprietary SaaS solutions. In this article, I’ll compare some of the best open-source and proprietary machine learning model monitoring tools available today.
MLOps
In an insightful session presented by Enrico Rotundo, we explore the innovative approach to scaling StableAudio globally. This presentation sheds light on the synergy between NVIDIA Triton and AWS SageMaker.
MLOps
Dr. Phil Winder shares experiences of Winder.AI’s MLOps consulting experience at a variety of large and small organizations. Abstract In this talk he presents industry observations of MLOps team size and structure for a range of business sizes and domains. Learn more about how others structure their MLOps teams. Discover which problems you need to solve first. About This Series Welcome to Winder.AI talks. A series of free interactive webinars hosted by Dr Phil Winder, CEO of Winder.
This page provides answers to our most common questions. If you have a query that isn't covered, please get in touch.
Machine learning operations (MLOps) is a combination of processes and systems to improve a model’s quality of service. This involves: authentication and authorization, logging, evaluation, explanation, operational maintenance, ownership, support, disaster recovery, monitoring and alerting, automated testing (both data and model), auditing, schema management, provenance, scalability (including to zero), model artifact lineage and metadata, governance and more…
Governance allows organizations to manage and control risk throughout the ML development lifecycle. Audit trails show which models are used to evidence that a model has been signed off for production deployment. Banks are good at this form of MLOps because they have regulatory requirements that force them to do so. But organizations everywhere can leverage the same techniques to reduce risk.
Provenance is often described as the ability to track a lineage, from a deployable artifact to the data it originated from. But delivering provenance provides robust, repeatable pipelines. Provenance promotes DevOps and GitOps—proven cloud-native techniques. And provenance provides uniformity – you’ll find that common operational patterns are reused because of the uniformity.
Operational automation helps reduce the toil involved with running ML models in production. This idea is less general and more specific. Precisely what you automate and how you do it depends on various non-functional requirements, like the size of your team or how popular the services are. But the benefits are universal. If you can automate a dangerous or tedious part of the process, this reduces the risk of mistakes, enforces compliance and decreases the operational burden on engineers and data scientists.
The team at Winder.AI are ready to collaborate with you on your mlops development project. We tailor our AI solutions to meet your unique needs, allowing you to focus on achieving your strategic objectives. Fill out the form below to get started.